
- Home
- Technology
- Is AI All Hype Or The Future? With...
Is AI All Hype Or The Future? With Siddharth Pai & Nitin Seth
A survey released by KPMG says that revenue generation has overtaken productivity as the primary gauge businesses use to measure AI's return on investment
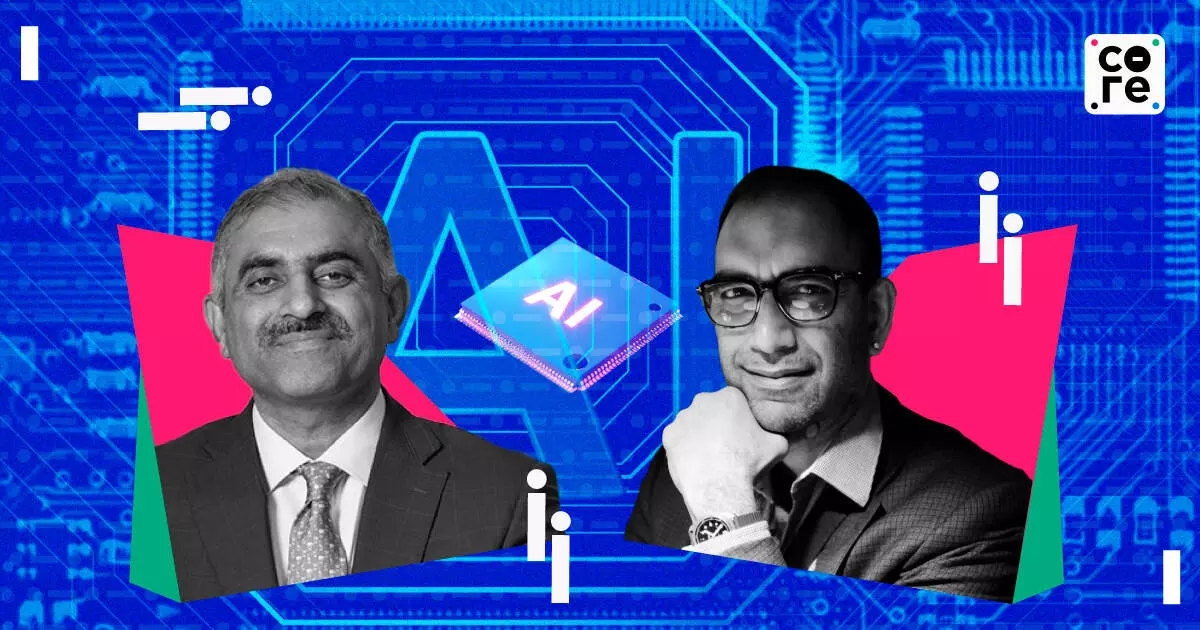
Chief technology officers across industries have been spending a lot on generative artificial intelligence, specifically on artificial intelligence in general. The hype around AI is visible in some ways from the way chipmaker Nvidia’s stock prices have soared in the last year – growing close to 800% since the start of 2023.
Nvidia chips are, in turn, going into computing in the form of data centres. There is a very strong underlying demand for these chips, which in turn represents the strides that AI is making. But some key questions arise here. Firstly, how much AI do we really need at this point in time? And how much AI have we been able to already use and if so, in what form? To what extent are we solving real problems with AI?
A survey released by KPMG says that revenue generation has overtaken productivity as the primary gauge businesses use to measure AI's return on investment. The professional services firm surveyed about 100 US based C-suite companies and business leaders, all worth about a billion dollars and more. On the other hand, organisations are expected to spend about $39 billion in 2024 on generative AI alone.
Chief intelligence officers are already talking about AI's efficiency savings, including a boost in productivity for software developers. Amazon, for example, said that it's using AI in a whole bunch of areas, from fraud detection to interpretation of rules and regulations, even validating invoices.
On The Core Report: Weekend Edition, financial journalist Govindraj Ethiraj dived into this with two guests — Siddharth Pai, technology columnist and founder of Siana Capital, and Nitin Seth, founder and CEO of Incedo Inc. which supplies AI solutions.
Edited excerpts:
Sid, why don't you kick off as someone who's a little on the outside and talk about where we are in the AI evolution today?
SP: I'll try and cover that on two or three different vectors. The first vector is the fact that AI is only now coming into its own. You know, the first attempts at having AI are arguably at least 70 years old, certainly at least 40 years old, when people in Carnegie Mellon, Cambridge and Oxford were coming out with computer based algorithms, which were mirroring the mathematical algorithms that could help with predictive AI as we know it. Of course, while the algorithms existed, the compute power didn't exist and there wasn't enough data for them to actually operate on to come up with worthwhile predictions. That since has come into its own… So that's one vector which is the technical vector.
The second vector, from an India perspective, is when you look at the fact that most of what happens from India is [exported] into the US and to other markets. The US represents about 55% of India's export services market, the UK and other countries. Interestingly, from a services perspective, whether it is IT based, or process based, or voice based, which is the last in the list, typically tends to be work that is repeatable, predictable, and just tedious to do. And in the past, [it was] useful to use India as a mezzanine step, or outsourcing, or offshoring as a mezzanine step, whether to India or the Philippines, because of automating, some of the tedious work was larger than outsourcing it or offshoring it. Now, we are coming to a point where the efficiency and the cost of actually getting this work done is lower than hiring people in India or the Philippines or elsewhere. So it represents a threat for the Indian workforce, especially the younger workforce, that's coming in without differentiated skills in either IT or in business, or in the specific processes we're talking about.
So I think on two fronts – One, we've had a technological leap, and two, there is a large population of Indian outsourcing employees whose jobs are at risk.
Nitin, you're in the space and your company is an AI company, so to speak, and you work with a lot of clients and you're solving real problems for them. So walk us through how you're seeing this.
NS: See, there is what Siddharth said, that AI has been in play for quite some time, so it's not a new thing. But over the last 2-3 years, we have seen some kind of a tipping point. And I think that was really triggered by generative AI — kind of letting the genie out of the box. I think there is both the substance of it and then there is the hype of it. The substance of it is that when you look at AI use cases, they have always been around, but largely…It has been very difficult to really make progress on them because of lack of data. So data is the core foundation for AI.
Now, Gen AI is helping solve that to an extent, because Gen AI is not based on a specific data set. It's based on the data of the world. So that is kind of a very big leap, you know, in terms of problem discovery, problem identification, a lot of problems which have been there. Now, using various GenAI methods, you can get a 40-70% initial answer, which to my mind is a huge breakthrough. And it's kind of led to a lot of very, very substantive relook at use cases. I don't think these are new use cases.
The second is the hype of it, that with OpenAI putting ChatGPT in the hands of individuals, it has led to that B2C explosion. And that's kind of putting pressure on the enterprises to follow suit. And there is just a very, very, very significant gap between the B2C adoption that we are seeing and on the more internal or B2B front. So I think that's the headline.
Now, in terms of the actual use cases, you can broadly say they are external and internal, but really kind of four categories. You have those which are impacting the customer experience and personalisation of some sort, which we already see. You know, you go to Amazon, you see personalisation, go to Netflix, you see personalization. So that the customer experience use cases have been happening are, you know, this continues to be a tremendous amount of work on that.
Then the second is how do you translate that into revenue?. And which is not through cross sell, upsell, churn management, new products, etc. I would say the impact on that is still limited. The experience is being impacted in a significant way, but the revenue impact is still not that significant.
And then the third vector is about efficiency, about automation. Siddharth also talked about automation, and there is a lot happening there. And the promise is so significant and at this point…I see an epidemic of POCs or Proof of Concepts. But the promise of the software development lifecycle, you can take 20% to 40% off that, not just in seemingly more routine processes like testing and quality assurance, but even in things like code reviews, code design. So that whole back office, mid office processes, all the technology processes, there is a tremendous, tremendous activity happening there.
There is also a lot of top down pressure from boards on this one. This is not CTO or CIO driven by any stretch of imagination. It is a top down agenda, if I may put it that way. But again, realisation of that, I think we are still in the early stages of that. At this point, the dollar spent on AI and Gen AI by enterprises is significantly more than the benefit they are getting from it. So we are still at that point in that entire cycle.
And the fourth use cases are really around risk and fraud detection, which are, there is a lot happening. And I think, at least in my mind, that's the only way you can solve for risk and fraud type of cases. Unfortunately, in that space, the attackers tend to be even smarter. So they're also using AI. So it's kind of like an AI war in which both the attackers and the defenders are using AI.
But look, overall, I think we will do a great disservice if we see this only as a hype or, you know, hey, this is something which needs to be limited or constrained in some fashion. The level of activity is absolutely tremendous. Maybe there is a question that, you know, can that level of activity be more thoughtful, more directed? So that is tremendous. And it is for a reason that the potential is ginormous, but the actual impact realised today is certainly less than the promise.
When boards are saying that, let's do something with AI, are they just saying that we've been reading about AI and let's go find something to do with it, or are they looking inwards and saying, okay, we've been grappling with, let's say, a resource mismatch and we want to rationalise resources? Or is it something that, okay, our competitors are doing it, and therefore we need to do it. So what's the driving motivation and to solve what problem at this point of time?
NS: Look, the board involvement is certainly what I see, and I mostly work with large enterprises and kind of big sectors like banking, telecom, life sciences. What I see is that the direction from the board is more than just high level. Okay? There is pressure. There are, you know, questions, targets being talked about. In most cases, most of my clients have to come and report to the board on a quarterly basis. There are at least a couple of really tech savvy people on the boards of most large enterprises that I serve. So it's a real pressure. Now, whether they have gone to the extent of saying that: Oh, here is a specific use that as a board we are sponsoring. Not really.
Got it. And how are you seeing it, Sid, from, again, maybe a little removed from the arena.
SP: Yeah, I got out of the arena.
And let me also add, if I can supplement that question – you've seen several technology waves, and you also advised clients on this in the past, and, of course, this is seemingly a bigger wave than many others. But again, if you were to contrast.
SP: I actually agree with Nitin. I actually liked his prior characterization of the four little windows…I think the push from boards, as he points out, is large. I still advise, largely friends. I just got back from a trip just late last night, where the entire discussion at a board level was around what Nitin was talking about.
With the tech savvy board… at least in my experience, I've seen there are specific use cases they talk about. For instance, they know that there are special areas in that particular company, which have been concerned in the past, especially on the cost side, or the efficiency side, or the technology side. And they hit on those quite hard. Uses for revenue building are still sort of up in the air. AI has always promised it. I mean, when I worked for the Carnegie group in the US 30 years ago, the main problem we were trying to solve was that of upsell and, and cross sell and so on and so forth, by coming up with recommendations.
While AI-used to try and solve that, and still trying to solve it, I don't quite, as Nitin said, I don't quite see solid uses around yet. The cost takeout use cases and the efficiency use cases are a lot more solid. So I agree with what Nitin said. I think at CIO level, the CTO level, what they're getting, and the CEO level, to be honest, is the push that they're getting from their boards is to be ahead of this technology, because it's a fundamental change in the way that we will be conducting business. The waves in the past were smaller, and you could afford to be a little late to the party because you could just have momentum take you forward. You can't afford to be late to the party. So I think that's why boards are scrutinising it as much as they are. So, yeah, I agree with everything that Nitin just said.
Let's look at some of the flip sides of AI as we know it. We know that there is a data bias problem. The initial push was to use all the data out there in the world and then said, no, that's dangerous. Let's focus on the data within organisations. And I think that transition is happening. But even within that, there is the possibility of data bias. There's of course, the famous term hallucination, where AI is not interpreting properly. How are you seeing that, Nitin?
NP: It's a massive, massive issue, Govind, and it's a massive area of activity. And frankly, that's where the majority of that [AI] spend is going on. And one is Nvidia on the compute infrastructure, and the second is on the data infrastructure.
There are four types of data problems. The first problem is, you kind of talked about it, which is, but I will put it differently, it's about contextualising the data. And I would submit that it's not about either or, Govind. It's not saying that, oh, you know, it's all Gen AI and the data of the world, or it's enterprise data within. You need both, let's be clear about it.
The second problem is the data integration problem. How do you bring it together? Because these are fundamentally very different. These are not even apples to oranges. This is like completely different planets. How do you integrate that data? You can't do it physically. How do you do it logically?
Then the third question, once you progress on data integration, is the data quality question. That garbage in, garbage out. We always know that. Now, the challenge that is happening, Govind and Siddharth, is that it's not just about the traditional measures of data quality, about accuracy and timeliness and blah, blah. It is also about context. In a particular context, a particular type of data may be okay. In other contexts, it is different. So the data quality question is becoming more complex.
And the fourth is about data security, which is, at this point, especially if you're in a regulated industry like financial services or healthcare, they have a slightly different concern, which is about personal data. Data security is a huge issue. And how does your data flow work? Because the nature of AI is that it's a bi-directional flow that you're getting the learning benefit. But what are you contributing back to it? How do you protect that? So those are the four issues.
You wrote this book called Mastering the Data Paradox, which I guess is sort of a prelude to some of the questions in the context of AI. So are these two linked? And, you know, good data, mastering the data paradox leads to better and more efficient application of AI?
NS: Absolutely. You know, I see data and AI as completely linked. They're conjoined. You know, they are both a cause and effect of each other. I think it's kind of, you know, futile trying to disaggregate them, because AI is the foundation of that is data. But then also AI, you use to solve for some of the bigger data issues. So, for example, the four issues I talked about, the data quality issue and the data security issue, actually, the way to solve it is also AI. So it's kind of like a Janus. It's like a kind of a double two-faced kind of a thing. And mastering the data paradox is simply saying that on one hand, we are having a data deluge, which is very difficult to deal with. On the other hand, there is an insight drought. There isn't enough to actually use.
So that's the data paradox. The drought, you know, the delusion drought—kind of like water, water everywhere, yet not to drop, to drink. And data is absolutely the unsolved problem. The compute is there. There are three things that go into AI—compute, algorithms, and data. The compute is there; it is just very expensive. So that has to be worked on. And that's a very big challenge for us in India.
Algorithms are largely there. Okay, there's a lot of progress that has happened. Off the three, the big kind of place where we are stumbling is data, because while the data volume and everything is there, but being able to sift out and say, okay, what is useful but, you know, what is actually junk, but still is kind of getting a lot of capacity and kind of cost, and we cut that out. I think those are the types of problems that we are still struggling a lot with.
Got it. Sid, can I come to you on the sort of data policy question now? We are using AI. We've adopted it. Governments are also looking at AI closely, whether it's the Government of India, the European Union, United States, everyone will have regulations of some sort. It may or may not impact enterprises and what they're doing inside those enterprises, but it'll definitely affect the way we use AI, at least as consumers or our data gets taken, which in turn may go back and affect enterprises. In your sense today, what are the key issues that we're looking at when it comes to, let's say, the way AI policy is being framed and should be framed in order to make sure that AI is more equitable in its applications?
SP: I'll tell you what's been happening so far is certainly with respect to data, what Nitin brought up earlier, which is personal data being safeguarded, has been the main factor on which governments are regulating.
Cybersecurity, huge, by the way, which is also, we touched on it earlier as we were talking about stuff and the ability to get to data which you shouldn't be able to get to with bad guys or the bad actors, which can be state actors as well, you know, is so much easier in this world because we're computing all over the place. There's just today's instance with Azure being out. There are flights that are even in India, people are having difficulty getting on the flight. Some startled media houses. I just wrote an article, just finished up one for next week for Mint. They were having trouble. They weren't the only ones. Others did. Anybody with a Microsoft-based system has been having trouble today, which doesn't bode well for Microsoft, and their data is all over the place.
And my classmate Thomas Kurian, Google Cloud, my old school classmate, is just recently in the news for trying for $26 billion to make Google's largest ever acquisition of a company called Wiz in the US again for cybersecurity. This is to make sure the data is safeguarded.
The regulations around keeping data local and keeping data within a country is going to become much more important, thereby blunting some of the efficacy.And the second thing is I think we will see a lot of bad state actors as well. There's one more last aspect that I wanted to switch on on data, which Nitin sort of covered, but there's nothing to do with policy, with the nature of the data and why Nvidia is doing as well – is that if you look at the data that's required for AI operations, especially things like Gen AI, where you're not working just on two variables, you're not working on an X and Y, you're working on a third variable, a Z or A, B, C, or whatever it is, the situation where your data is not in tables, they're not in rows and columns, you also have another vector, which is the depth.
And the reason why Nvidia is doing as well as it's doing is with GUI chips, they have the ability to deal with data in three dimensions rather than just two dimensions. And that is another thing that increases the complexity of the kind of data that we're dealing with from an AI perspective. So I think, you know, hopefully we have chip manufacturers or at least chip designers who can get to the same level that Nvidia is at and give them a little bit of competition right now, because the monopoly at this point is frightening.
The rents are huge, you have to wait two years or more to get computing capacity, and if you're in India, it's probably longer, as Nitin pointed out. And, you know, hopefully there should be some policy work that breaks up really soon, I hope.
Thanks for that. We're running out of time. Nitin, two things. One is, how are you seeing AI policy evolve and when I say, or rather policy governing AI? Second is, from what you've seen in the last couple of years and the kind of investments that have gone in, what would you tell companies, boards, CTOs and so on today? Not just in terms of investments, but the next layer.
NS: First part, you know, the AI policy, I don't really see it as different from the data policy. I think this is a very joined up debate. I really, I'm very concerned about it, I'm very concerned about it because of two reasons. First is that this is something that really needs global standards. It really needs global standards. It's kind of like, you know, global emission standards. And you can have some country specific things, but you need a basic standard because this is not a local play at all. You know, this is, you know, data privacy. You know, these are not choices that you can get individual consumers to be making. It's too complex for them. You need to have a basic standard. And today there is almost no service, which is purely local. So there is a need for global standards that is not happening enough and not at the right level.
The second issue is that because these issues, you know, data privacy and data security, these are the big issues. See, bias is going to get addressed. But data privacy, which is at an individual level, data security, which is at an enterprise level, these are mega issues. They can, if there is something which can bring down the AI revolution, it is these two. Okay. It is these two. And, you know, and like this whole Microsoft outage, it kind of, you know, brings, you know, reactionary, large, you know, reactionary things, right.
And it kind of really brings that governance. And what I also see is that, especially the banks, that they have not made a lot of progress compared to some other sectors and compared to the data they have. And I think it's because, you know, that governance, you know, is stifling innovation.
So there is this trade off, you know, security and privacy are extremely paramount. You need to handle it in a very mature fashion to kind of release the power of AI. But if you're not handling it well, it can actually, you know, block this entire thing. And, you know, bring this again, the heavy hand of, you know, governance, you know, both internal and external. And, you know, and I think that's a very big challenge. And today, the level of seniority, the level of maturity that is needed in the debate is not there. Because whenever I am in data policy, you know, conversations, they're all with a very restrictive perspective.
Now, on your second question, you know, what do I tell companies? Look, AI is a bandwagon you can't get off. You have to do it. So all this thing about how much is enough and should you do it? Don't get caught into existential debates. You have to do it. You have to do it. But you have to do it smartly. Just don't go with the hype.
One of the first questions that you ask, that you have to be specific about the problems you're looking to solve. Those problems at the end of the day come largely from your customer problems. Be specific. If you're specific, I think it will allow you to direct your AI efforts a lot more intelligently in a more focused fashion, because the two places you're really spending money are on your compute capacity and on your data.
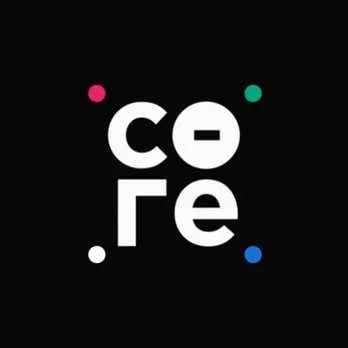
A survey released by KPMG says that revenue generation has overtaken productivity as the primary gauge businesses use to measure AI's return on investment

A survey released by KPMG says that revenue generation has overtaken productivity as the primary gauge businesses use to measure AI's return on investment