
The Positive Impact of AI on Our Lives with Ravi Bapna and Anindya Ghose
30 Dec 2024 6:00 AM IST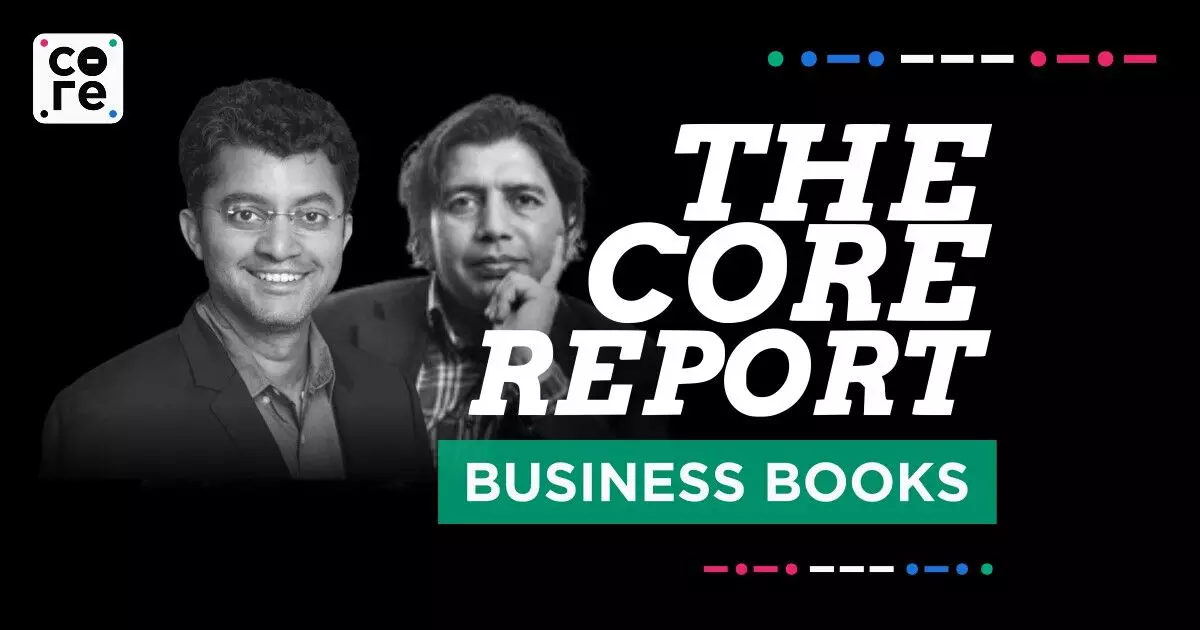
In this Business Books edition of The Core Report, financial journalist Govindraj Ethiraj sits down with professors Ravi Bapna and Anindya Ghose to explore the multifaceted impact of artificial intelligence (AI) on education, misinformation, and global technology trends. Their discussion highlights how personalized AI tutors can revolutionize learning, the collaborative role of AI and humans in tackling misinformation, and the geopolitical implications of advancements in the AI technology stack.
From India’s strides in digital public infrastructure and AI for regional languages to the dynamic competition in the semiconductor and cloud computing industries, the conversation provides a forward-looking perspective on how AI could shape 2025. Bapna and Ghose also address the challenges of AI adoption, emphasizing the need for educators and organizations to adapt to rapidly evolving technologies.
Tune in for insights about the transformative potential and ethical dilemmas of AI in our interconnected world.
NOTE: This transcript contains the host's monologue and includes interview transcripts by a machine. Human eyes have gone through the script but there might still be errors in some of the text, so please refer to the audio in case you need to clarify any part. If you want to get in touch regarding any feedback, you can drop us a message on [email protected].
—
Govindraj Ethiraj: Ravi and Anindya, thank you so much for joining me on this special Business Books edition of the Core Report. So we're going to talk about Thrive, maximising well-being in the age of AI, the book that you've written and worked upon for some time. Now before we get into some specifics, a slightly or rather a bunch of broader questions.
First is, the sense I got from the premise that you're starting the book on is that people believe that AI is causing harm in some way or the other, or maybe more of AI causes harm or is perceived to cause harm, and therefore you felt the need to address it, which is the perception of harm, and point out therefore all the good things that it does. So would that be a fair starting point?
Anindya Ghose: Yes, first of all, Govind, thanks for having us. I would say that's a fair point, and to just give you some context, both Ravi and I have been working hands-on in the AI space for almost 20 years, doing research, teaching and consulting. And in the course of all of that, we basically kept hearing a lot of fear-mongering and backlash against AI.
And I think part of that was essentially promoted by mainstream media or other kinds of media to kind of, you know, fear cells, right? Hope is not as attractive fear cells. And so both Ravi and I thought, hey, you know, we felt compelled to sort of change the narrative or at least talk about the other side of AI, which is that AI has already had a lot of positive impact on some really fundamental aspects of human life.
And, you know, we have our own research, we have a body of research from academia to support all of that. And so the genesis of Thrive is that rather than having this very extreme dystopian or utopian narrative about AI, we said, you know, we're not dismissing the negatives, but no one's talked about the positives. So there was a need for us to, or for someone to, you know, sort of talk about the other side.
Govindraj Ethiraj: Right. And you've used a few illustrations and you've used it, for example, in the context of dating apps or in the context of Airbnb. Now, and of course, you've also used it in the context of health and medicine.
And we'll come to that in some detail a little later. But tell me why you picked up this. Is it because this is where people encounter AI the most when, let's say, looking at a good looking house on Airbnb, which you've talked about, or let's say using a Bumble or a Tinder, which of course is not in India, but I guess many who would be watching this or hearing this would know what it is.
Ravi Bapna: So, well, thanks for having us, Govind. And I think just to sort of, you know, take a step back and emphasise that in comparison to prior general purpose technologies, right, you think about, you can go all the way back to the printing press or the steam engine or the computer or electricity. Right.
Those were all very tangible. Right. So I think the reason why this kind of, you know, I guess, fear mongering narrative is allowed to persist is because AI is more complex.
It's intangible. It's layered. These algorithms are doing, you know, things in the background.
For example, you know, influencing who you date on Tinder or who, you know, what kinds of listings you see, even in an advertising scenario, you know, let alone in, you know, Airbnb kind of platform. And of course, you know, lots of very interesting applications in healthcare and so on. So we had two choices.
You know, both of us on business schools, we could take the completely corporate route where we could give examples of how companies are using this. But because we wanted to try to demystify AI for everybody. Right.
So literally like the layperson, we thought it would be more interesting to talk about things that everybody cares about. Right. So everybody cares about your health care, your work, your education, you know, who you meet, where you stay.
And that was the reason why we chose that direction for the bulk of the book. And those were the examples that we wanted to pick up on.
Govindraj Ethiraj: And walk us through some of those examples. You know, one thing I thought was interesting was that, you know, for example, the concept of having good looking pictures on Airbnb or for that matter, I guess, when you want to sell a house. But at the same time, while the platform is using AI to sort out and maybe to create some kind of hierarchy, the user too has access to AI in some way to actually select what she or he wants.
And I think the example that you use of cameras.
Ravi Bapna: Yeah. Yeah, absolutely. So I think, you know, there is a lot of AI models now that, as you know, can handle, you know, multimodal data.
Right. So not only just textual data, numeric data, but image data, video data as well. And so the research that we point to in the book is actually done by some, you know, friends and colleagues at Carnegie Mellon.
What they found was that if you could, you know, they could actually quantify literally the impact of improving your photo quality. Right. In terms of listings.
And as well as in the end, you know, both in the dating world and also in Airbnb, what we are fundamentally in is a matching problem. Right. It's a job of matching, you know, preferences of two sides.
And there is a lot of, you know, backend algorithmic innovation that, you know, tries to get these matches to work in a way that maximises overall welfare out there. Right. And so I think these matching algorithms are not, again, fully well understood.
But, you know, they play a role literally in, you know, so many settings. And I think those were the examples that we wanted to pick on. And the interesting thing is now that in the past, we could only consider like numeric attributes.
But now we can actually deal with, you know, much richer data. Right. And that, you know, changes the whole game in so many ways.
Govindraj Ethiraj: Can you illustrate that, Ravi, when you say numeric to dealing with large sets, whole sets of data?
Ravi Bapna: So, for example, you just think of, you know, like text. Right. So, if you go into a typical dating scenario, right, if we just had to look at a few attributes, you know, we can only make a certain quality of matches.
But if we can get broader into, you know, looking at what you write in your description, both in just the actual words, also in a semantic sense, as well as if we can look at, you know, the images that you put out there, then that creates a much richer feature space. Right. It's now I'm looking at, you know, I can match two people based on 200 dimensions as opposed to on 20 dimensions.
Right. Otherwise, you know, think about like, you know, Airbnb kind of setting. You might look at, OK, you know, I want to look at the size of the house.
I want to look at, you know, the number of bedrooms, the number of bathrooms. So, those are purely quantitative features. Right.
But now I can get into more latent preferences that people have that can be richly expressed in text and images. And that allows richer matching, basically. And I think lots of studies have shown that this increases the overall welfare, like both sides win, actually, in some sense.
Govindraj Ethiraj: Right. So, you know, you talk about, you've talked about matching and matching obviously links to bias and then harm. Now, that's something that you've talked about in your book as well all through, including in healthcare.
And I'll come to that again a little later. But tell us about what are the advances in tackling harm in the areas that you've seen harm and what are the issues when it comes to bias and some of the examples that you've quoted or have worked upon and what's changed even in this period that we've been talking about it or let's say between the publication of your book and now.
Anindya Ghose: So, I think, you know, the first thing I would say is that there's been a lot of advances in ensuring fairness in algorithms and AI. And associated with fairness is the notion of de-biassing. So, in the simplest sense, de-biassing essentially means that, you know, the data scientist takes looks at the data set and tries to find certain outliers that might be driving the decisions.
Or another form of de-biassing is that to identify whether the input data itself was, you know, fraught with, you know, some sort of selection issues which lead to the algorithm or the recommended system giving you all kinds of one-sided recommendations. So, that's basically bias, right? The bias means that certain demographics may be benefiting disproportionately from a certain AI algorithm.
And the good news is, and this is something that, you know, we've both like done hands-on work, but we also teach to our students that de-biassing is actually not rocket science. It's fairly straightforward, right? Anybody with, you know, some descriptive or statistical background should be able to find these outliers.
And so, the good news is, you know, we can actually have solutions for de-biassing based on everything that we have been seeing. And in some ways, you know, the solution to the bias in AI is actually more AI. So, in other words, we can de-bias the biases in AI using advances in AI.
Ravi Bapna: So, just to give you a couple of examples, you know, so, you know, the classic story that was in the media with Amazon when they used the resume screener, you know, the early, the first version of the resume screener that Amazon came out with was called out in the media because it was basically, you know, discriminating against women for tech jobs, right? And I think the reason for that is all the reasons what Anindya was mentioning, you know, the source data itself, because of historical hiring practises, you know, the training data did not represent women adequately. And therefore, machine learning, which is basically learning by example, right?
It's like how kids learn. That's how machine learning actually works technically, you know, is not able to learn that well, right? So, it's more likely to make mistakes.
It's more likely to have, you know, a higher rate of false negatives or false positives for a subgroup of people. In this case, it was women, right, that were basically, you know, it was, you know, it was giving more false positives compared to men. And therefore, they were not being hired.
So, now the solution to that, as Anindya said, is, you know, using, you know, techniques like reinforcement learning that will say, you know what, yeah, I have a ranked list of candidates, but 10% of the time, 20% of the time, I'm just going to strategically explore, right? So, think about explore and exploit as baked into the algorithm. So, I'm going to take a chance on this other candidate who is not scoring the highest in my algorithm right now, but I want to learn more about that subgroup, right?
And that turns out to be a really, you know, powerful way to actually, in the context of hiring, to debias, you know, the approaches. And there are many other examples. You know, we can talk about, you know, for example, in the summer, we were working with the Mall of America.
They were deploying facial recognition technology, right? And this is something that people get really panicky about because, again, it has been shown. There have been studies that say that, you know, the false.
So, the reason to deploy this is to prevent bad people from coming into a public facility, right? People who are banned, let's say, for prior reasons. Now, if the false positive rate, again, is different by different subgroups of people, right, then you could have, you know, a case of wrongly accusing somebody of being, let's say, you know, a criminal, and you would stop them.
So, we had to educate the leadership in deploying the model in the right way, choosing the right thresholds, so that they can minimise the false positive rate for the subgroups at the expense probably of, you know, maybe making a few more false negatives, right? So, you let maybe two other bad people in in a month to move your false positive rate from, like, one in 10,000 to one in a million, right? And that can be done.
Govindraj Ethiraj: Right. So, you know, this is also about analytics and the whole process of building that intelligence into the system, which allows the system to then, you know, spot, look at past data and then obviously try and figure what could happen. So, you've described it as descriptive and predictive and causal and prescriptive.
So, I mean, the sequence seems pretty clear to me. But could you walk us through how this goes in the mind of a computer or inside a computing system? And what is the manifestation outside?
And what's a good example of that?
Anindya Ghose: Sure. So, I would say that, you know, we think of this as a framework that would help organisations who are embarking on this AI transformation journey. And so we start with a very, very simple descriptive pillar.
And the pillar is essentially helping organisations answer the question, what has happened so far? Okay. So, before you do any sort of modelling, just tell me what has happened so far.
Right. And an example of this could be like a clustering technique. Are you trying to segment your customers in a cluster so that you can scale up the intervention that you're trying to design?
The second kind of pillar is predictive. And that's basically asking the question, what will happen next? Okay.
And there are lots of example predictions. So, you know, we basically looked at advertising, target advertising, an example of, you know, who will buy a product based on what ads they're being exposed to. Can the algorithm help predict, you know, how responsive the targeting would be?
Right. The third question is causal. That helps the organisation answer, why did something happen?
Okay. And this is an incredibly important and, yes, obviously, notoriously difficult question to answer. But every organisation, we believe, should be thinking about causal influence, too.
Because, you know, unless you can actually understand the reason why something happened, there's no meaning or there's no point in scaling up the intervention that you're trying to design. And then the final pillar is what we call prescriptive. Prescriptive essentially says, what should I do next?
So we help companies in this framework. We help companies go through these four stages. What has happened?
What will happen next? Why did something happen? And what should we do?
And that gives the, you know, the sea-suit folks the entire armoury of ammunition that they need to deal with, helping transform the organisation in whatever stage. This can apply to, you know, a Series A startup company to a Fortune 100 well-established company. This framework is industry agnostic.
Both Ravi and I have deployed this in dozens of industries, in dozens of countries. So, you know, we really feel good about this framework.
Govindraj Ethiraj: Got it. So, you know, when you talk to companies or rather when companies talk to you, are they clear about why they want AI today and clearer today than compared to, let's say, even a year ago? Or are people saying, I need, everyone's talking about AI, I need to do something with AI.
So come over and, you know, do something for me.
Ravi Bapna: I think it's more of the latter going, you know, you hit the nails on the head. You know, and unfortunately, what's happened is with Gen AI, that's the new shiny object in town. You know, the myth now is that everything is about Gen AI, actually, right?
And in fact, you know, we are very confident that 70 percent of the value in the next, you know, three years is going to come from some of the core pillars like prediction, right? So if you're in health care, can you build models using the data that you already have to detect disease, you know, two months before it is likely to happen, right? And can you intervene with that?
And, you know, lots of examples of that as well. So it tends to be unless you are, you know, a handful of companies in the world, you know, maybe we can point to 20 companies where they understand every component in the pillar really well. They know, you know, when to use reinforcement learning versus when to use perhaps deep learning.
Right. I think the vast majority of companies are really, you know, kind of scratching the surface at this point. And it has to a lot of this has to do with actually educating the leaders.
Right. So I think for us, you know, that is a big source of our efforts and time that we spend because we feel that we feel that it has to start from there. Of course, we train data scientists as well.
So, you know, both at NYU, at Carlson, we have very robust MSBA programmes that, you know, Anindo runs it there. And, you know, I've been a co-designer here. But at the same time, it's not enough to create the supply of data science and, you know, AI talent.
We also need the demand from the leadership. Right. Because they have to understand the different components and then fund projects in the right way, prioritise.
So we see, unfortunately, a lot of phishing, but I think the smart companies know that they need to get help and then they reach out.
Anindya Ghose: Yeah. One thing I would add to that, Govind, is that the flip side of all this is that there is something called snake oil. And, you know, when we when we work with companies or when we are asked to evaluate, you know, portfolios of companies, you know, recently there was a private equity firm who was investing in a bunch of AI companies.
And, you know, they asked us, can you help us evaluate how good it is? And a lot of the companies that were claiming AI were basically running a logistic regression, not AI. And so I just want to make sure that, you know, your audience understands that there is snake oil.
But that's where, you know, if you work with the right people, if you have the right framework and the right tools, you'd be able to distinguish what is bona fide from what is snake oil.
Govindraj Ethiraj: Yeah, let's go to healthcare. And, you know, you said that basically there are some good use case examples in healthcare. And we've seen some, including in India, of, let's say, analysis of chest X-rays or pulmonological efforts or initiatives.
So tell us about the kind of work that you've been seeing and the application of AI and what is it doing in terms of better diagnosis or better, faster diagnosis?
Ravi Bapna: Yeah. So, you know, one of our favourite examples in the book is from the Mama Clinic out in Budapest in Hungary, where they trained a deep learning model to look at essentially mammograms. And again, so radiology has been a great starting point because there's been a lot of digitised data available in radiology.
And again, you know, the underlying fuel for AI is data. So we need those historical cases of diagnosis of, you know, yeah, this particular set of mammogram images has malignant tissue and this does not have malignant tissue. And so there, you know, it was really heartening to see that there were actually, you know, 22 instances when, you know, trained radiologists and oncologists actually missed out on detecting cancer that the AI caught.
Right. And so in that sense, you know, the future really going forward is this AI plus human augmentation. And in fact, I was on a panel earlier this summer with the head of AI at Mayo Clinic, you know, which is a renowned hospital right here in Minnesota.
And, you know, they were kind of, you know, categorising the use cases into three buckets. Right. So there are high risk use cases with no hospital is going to get into right now.
And no hospital is going to ask a doctor to, you know, use AI to do diagnosis directly. Right. But, for example, let's say a patient walks in with a, you know, 5000 page patient chart.
Right. And most of the people who go to Mayo Clinic have unfortunately very complex conditions. That's why they are there.
AI is really good for synthesis. Gen AI in particular is amazing for synthesis. So, you know, the doctor only has 15 minutes to prepare for this upcoming visit with this really, really complex case.
So they have already built applications where generative AI can synthesise the key issues that the doctor needs to focus on. And, you know, this person is really much, much better prepared for a meeting. Right.
They also have other use cases where they're looking at, you know, EKG data in the context of cardiac disease. And, you know, they've already successfully deployed models where they can actually, even though to the naked eye, like to the not to the naked eye, to the trained cardiologist, they can't pick up a pattern right now. But when you look at, you know, historical data combined with other socioeconomic indicators and other factors, you know, a particular pattern in an EKG they found is going to be predictive of, you know, some kind of heart disease, like maybe, you know, two months from now, not right away.
Right. So I think that's where it gets exciting.
Anindya Ghose: Can I give you an example, Govind, on health care?
Ravi Bapna: Yeah.
Anindya Ghose: This is a close up to what most people will probably identify with. So, you know, we have these wearable devices and fitness tracker. We all wear these bracelets.
And people wear these bracelets for identifying their sleeping patterns, exercise patterns, maybe even nutrition. So my co-authors and I actually ran these large scale field experiments with platforms that have these mobile apps and wearable device trackers. And particularly if we focus on chronic disease patients, you know, patients on diabetes and, you know, blood pressure, cholesterol and so on.
And the idea was that we would, you know, randomly allocate these devices to patients, obviously, you know, to prevent any selection issues. And there would be an AI algorithm in the background that would essentially be monitoring all of your wellness data, your behaviour patterns, data, sleeping, exercise and nutrition, and then send you recommendations accordingly. And then you would have to act upon it.
And the punchline is that after a 15 month period, we saw a statistically significant improvement in the patient's outcome. So, for example, for diabetes patients, there was a significant drop in their H1AC levels, blood glucose levels, haemoglobin levels and so on. And so but the underlying engine behind all of this was this algorithm was essentially a machine learning algorithm, taking real time data and patients acting upon it.
So there's another very, you know, common example that we can see happening.
Govindraj Ethiraj: And you're seeing all of this scale up, Anindya. I mean, I know there are some good examples and good pilots and projects and so on. But where is it going next?
Anindya Ghose: Yeah. So the latter one. So, you know, the fitness tracker example, there is definitely a lot of scaling up.
I was recently talking with Vishal Gondal, who is an entrepreneur in India. He has this company called GoKey. Yeah.
Govindraj Ethiraj: He's right here in Mumbai.
Anindya Ghose: Yes. Yes. So I met him last month.
And, you know, we had this fascinating conversation where he's also doing something similar. Essentially, people who adopt the GoKey app will essentially be able to record their behaviour of endless exercise data. And then they can actually use that for e-commerce shopping.
So like, you know, his company takes it to the whole new level where you can actually basically share your data and return for these personalised benefits, which goes to another very interesting theory that, you know, in the context of privacy, right, you know, these companies trying to use your data against you and all of that. But there is this big segment of society that is very willing to share their data in return for concrete benefits. And I think companies like GoKey in India and many others around the world can really leverage that.
But the good news is the engine powering all of this is some combination of predictive and causal AI.
Govindraj Ethiraj: Interesting. And there are many more examples I'd love to talk to. But I think in the interest of time, let me pick on one, perhaps, and by extension, another one.
So education and the role of AI in education, that's something you both have spent time on in this book as well. And also as an extension, can you talk a little bit about coming back to the harm side and areas like misinformation and what you see the role of AI? But first, let's start with education, which is the positive side of it.
And how that can improve student-teacher interactions or overall student interactions and make the whole process better or more efficient or just more happy.
Ravi Bapna: Yeah, I'm glad you asked us about that because it's such an important area. And I think the punchline really is the ability now to personalise, essentially, education. So think of basically the personalised tutor example that we have in the book with Kanmigo.
But we have deployed that even in the context of business schools, in educating managers on how to become more data-driven. And the idea is that you have a tutor, just like you might hire a personalised tutor if you add a lot of resources. Wealthy families can do that for their kids, but we can't do this at scale.
But if you structure Gen-AI correctly with the right guardrails, then you could have two situations. Let's say a kid who's struggling with math maybe asks this tutor to solve a particular calculus problem. Hey, but the tutor is smart enough, it'll never give the answer.
It'll give you the next question to ask to get to the right answer right there. Or you could have another kid who's struggling with literature where the tutor will say that, oh, yeah, you're not ready to read this book. But maybe you can have a conversation with a character in the book.
And Kanmigo has deployed that in the context of early education. We have already started deploying this in the context of business education in our programmes. So I think this is going to really enhance the learning potential.
But right now, we need more awareness and more adoption of this approach from faculty across the board. So I think education, especially in this ability to personalise it in the right way and structuring it, we have to think about Gen-AI beyond just prompt engineering. We have to create these agents and we have to create these architectures like the RAG architecture that allow us to really create very nice solutions out there.
And with misinformation, I mean, Ando can weigh in. My view is that, yes.
Govindraj Ethiraj: Harmful content as a whole.
Ravi Bapna: Yeah, right. I mean, I think so, again, we have to get much, much smarter as a society and educate ourselves in becoming smart consumers of what we see out there. Right.
We should you know, we can't take everything at face value. Right. And I think there's going to be tremendous opportunities and players and entrepreneurship that is going to help us.
You know, quote unquote, let's say if it's educational content, let's watermark it, you know, in the right way so that that's credible. You know, maybe it's, you know, you know, maybe NASSCOM is the right authority, but we are going to have to meet two things, education and, you know, awareness on the side of the consumer, as well as, I think, help from the side, from the technology side. Anindar, if you want to weigh in.
Anindya Ghose: So I would say, you know, taking a step back, Govind, on the misinformation thing. So, you know, so we have this interesting world where there is AI, there's humans, and then there's AI plus humans. I think misinformation is one of the best use cases where AI alone or humans alone won't be sufficient.
We actively need the collaboration of AI and humans. Right. And so I think if you look at some of the larger examples of misinformation being a problem, like typically these are digital platforms that we know of.
Right. And the ones that have done a really good job. Right.
And are the ones who have done this combination of human moderators in combination with algorithmic training. So humans are still providing inputs to algorithms to figure out what is fake news is what is bona fide news. Because the algorithm on its own is not intelligent enough to figure it out.
So there are some cases where AI on its own is in great shape and it can be unleashed to harness a lot of potential. But I think, you know, misinformation and harmful content continues to be a use case where you need both human beings and AI.
Ravi Bapna: Yeah. And we can teach the AI to learn really fast from humans. So one issue that comes up is people say humans can't scale.
Actually, as humans make smarter decisions, AI is going to watch that and learn from that and that can scale.
Govindraj Ethiraj: Right. So let me ask you a slightly more horizontal question if that's the right way of putting it. So, you know, we are reaching the end of 2024.
This has been an eventful year for many reasons. And there's 25 coming up, which will be interesting among other things for maybe, you know, a new world order, at least on the business and trade and tariff side coming up, including in where you are in the United States. So that's one part of it.
What are you both seeing on in terms of technology trends and maybe in the context of AI? What do you think could be some of the things that could happen or we should be looking out for in 2025?
Anindya Ghose: So I'm glad you asked that question, Govind, because this is where economics meets geopolitics. So I would start off with, you know, the world of semiconductors. So if you look at the AI stack, right, the AI stack is essentially the Gen-AI stack is fundamentally four things.
Semiconductor chips at the bottom, followed by cloud computing, followed by large language models and followed by the application layers. Now, nothing will happen in the entire stack until you have the right set of chips. And what is now happening, at least for a while, is this bimodal, bipolar world between U.S. and China, the two countries leading in the AI arms race. But as you know, the U.S. and the West have essentially handicapped China from accessing some of the most relevant chips. So and this goes back to then the question of like what will happen next. So two things I mentioned.
One is up until recently, there was this credible threat of the Taiwanese invasion by China because PSMC essentially is the fabrication factory. And the U.S. and the West doesn't want China to have access to that. And China wants it.
But very recently, something very interesting happened, which is Huawei, the Chinese telecom provider, released the first 5G handset, the smartphone, with indigenous semiconductors produced in mainland, along with a non-Android operating system also produced in China. And that is now telling us that, OK, they have their capabilities in-house to be able to figure this out. And so maybe then the probability of the Chinese invasion of Taiwan goes down.
But it all sort of comes back to the world of AI stacks, semiconductors that are essentially creating all these possibilities.
Govindraj Ethiraj: Ravi, what are you seeing in 2025?
Ravi Bapna: You know, and I'll focus the lens on India. I think, you know, there's lots of exciting entrepreneurship happening. You know, we travel a lot.
You know, both of us have deep connections at ISB in Hyderabad and other boards of several companies. And, you know, so for me, I think, you know, the movement to create, you know, these large language models that are specific to Indian languages. Right.
I think, you know, and to put that out as a digital public infrastructure. Right. I think, you know, so AI for Bharat, Bhashani, these projects that are happening at IISC and Indian Institute of Technology in Madras, you know, funded by people like Nandan and others.
I think, you know, that model, the digital public infrastructure model actually is a model for the world. Right. I don't think the West has woken up to that yet.
You know, and that's why India has the most sophisticated payments network right now. You know, and if, for example, Gen AI, you know, on one hand, the stack that Anindu was talking about could actually, you know, there are a handful of companies that are there in all of those layers. Right.
And that that could again lead to lead us towards, you know, monopolies and things like that. But, you know, in India with identity, with payments, we, you know, we've understood and we've proved the digital public infrastructure concept. You know, I'm really excited to, you know, see AI come in in that form.
And I see a lot of opportunities for, you know, solving problems in, you know, in rural Bihar, perhaps, right, where people don't have access to, you know, maybe same level of sophisticated technology. They want to speak their own language. Right.
So I think for me, that's what I'm keeping my eye on right now.
Govindraj Ethiraj: Right. And last question to both of you. So as you look ahead, I mean, what's the one thing that you personally are excited about or looking forward to, which could be maybe like a game changer?
Not necessarily, but let's say could be. And the second question, I mean, on the flip side is that what's the equally big concern that you have as you look ahead? So question for both of you.
Anindya Ghose: I guess for me, you know, the interesting thing I'm looking forward to is this going back to the AI tech side, this competition that is now brewing across, you know, a few companies, but in each layer. Right. And so you see that, you know, if you asked me a year ago, like, can you imagine Amazon being the one of the most powerful or dominant gen AI companies having a presence in every layer?
Like, imagine Amazon producing semiconductor chips. It's happening. So they are producing Tranium 2, which is competing head to head with NVIDIA's GPUs and Google's CPUs and AMD's CPUs.
So, like to me, I find that very interesting that a lot of the discussion in AI is on the software consumer facing side. But personally, I find it very interesting to think about the hardware side, the semiconductor side or the cloud computing side. And I think that the fact that all these companies are again jostling for, you know, competition, dominance, power in each layer, I find that very fascinating.
Ravi Bapna: And, you know, my fear, which is actually also the opportunity, is again based on, you know, what we've seen over the last two decades working in this space, is that companies' ability to absorb these technologies actually is very, very limited, right? We are barely scratching the surface. Even if we take all the technologies that we have today and start using them, you know, you could significantly improve productivity, you could improve, you know, improve human welfare.
And so I think that as educators in business schools, you know, that also is an opportunity for us, right? And a big part of, you know, what I'm spending a lot of time right now, even in my research, is actually, you know, creating this awareness amongst, you know, business school professors and business schools around the world that our world is getting disrupted. And we have to teach in a way that is much more engaging, richer, experiential, and, you know, get students to tackle real problems in the classroom, as opposed to, you know, doing what we were doing five years ago, right?
I think those professors are going to get disrupted. You know, so I think, you know, to me, that's a big opportunity, because if we can get more people to understand this technology and use it in a way that can, you know, maximise the benefit and minimise the risk, then, you know, we can improve, you know, lives all around, essentially.
Govindraj Ethiraj: Ravi Bapna and Anindya Ghose, it's been a pleasure talking to you both. Thank you so much for joining me on The Core Report.
Anindya Ghose: Thank you for having me.
Govindraj Ethiraj: Yeah, real pleasure.
